Redes neuronales artificiales para la estimación de la socavación en pilares de puentes en el Perú
dc.contributor.advisor | Chávarri Velarde, Eduardo Abraham | |
dc.contributor.author | Delgado Caballero, Fernando Erdulfo | |
dc.date.accessioned | 2024-12-23T21:20:53Z | |
dc.date.available | 2024-12-23T21:20:53Z | |
dc.date.issued | 2024 | |
dc.description | Universidad Nacional Agraria La Molina. Escuela de Posgrado. Maestría en Recursos Hídricos | |
dc.description.abstract | La mayoría de los puentes colapsados han fallado por socavación en los elementos de apoyo. Además, la socavación es un fenómeno complejo, por lo tanto, la relación entre las variables que la definen aún no ha sido estudiada lo suficiente como para encontrar una función que produzca, en simultáneo, estimaciones óptimas desde el punto de vista técnico y económico. No obstante, está bien documentado que las Redes Neuronales Artificiales permiten encontrar patrones y/o tendencias, las cuales podrían ser utilizadas para realizar estimaciones. Por lo tanto, el objetivo de la presente investigación fue proponer una Red Neuronal Artificial (RNA) que permita estimar de manera eficaz y eficiente la socavación en pilares de puentes ubicados en la costa del Perú. Para alcanzar dicho objetivo se utilizó una RNA de tipo Perceptrón Multicapa, la cual fue entrenada y validada utilizando la base datos A Pier Scour Database del United States Geological Survey. Se construyó una RNA Base, de la cual se generaron tres versiones que se diferenciaron en el tratamiento que tuvieron las variables; seleccionando para ser optimizada la versión que tuvo mejor performance en función de la Raíz del Error Cuadrático Medio. Las estimaciones de la RNA Optimizada se compararon con las mediciones de socavación hechas en los pilares de los puentes Aramayo y Pisco durante el periodo diciembre 2021 – mayo 2022, y con las estimaciones hechas utilizando la ecuación del HEC – 18 para socavación en pilares. Se concluyó que la RNA Optimizada no pudo estimar de manera eficaz y eficiente la socavación en los pilares de los puentes antes mencionados. Aunque la RNA Optimizada produjo mejores estimaciones de socavación en los pilares de los puentes Aramayo y Pisco que la ecuación del HEC – 18, estas estimaciones de la RNA Optimizada distaron considerablemente de las mediciones de socavación hechas en dichos pilares. | |
dc.description.abstract | Most of the collapsed bridges have failed due scour around the supporting elements. Moreover, scour is a complex phenomenon, therefore the connection between the variables that defines it has not yet been studied enough to find a function that produces, simultaneously, optimal estimations from technical and economic point of view. However, it is well documented that Artificial Neural Networks allows to find patterns and/or trends that could be used to make estimations. Therefore, the goal of the following research was to put forward an Artificial Neural Network (ANN) that allows to estimate effectively and efficiently the scour around bridge piers located in the Peruvian Coast. To achieve this goal, a Multilayer Perceptron-type ANN was used, that was trained and validated using the database «A Pier Scour Database» of the United States Geological Survey. A Base ANN was built; three versions of this ANN were generated that differed in the treatment of the variables; selecting in order to be optimized the version that had the best performance based on the Root of the Mean Square Error. The estimations of the Optimized ANN were compared with the scour measurements made around the piers of the Aramayo and Pisco bridges during the period of December 2021 - May 2022, and with the estimations made by using the HEC – 18 equation for pier scour. It was concluded that the Optimized ANN could not estimate effectively and efficiently the scour around the piers of the previous mentioned bridges. Even though the Optimized ANN produced better estimations of scour around the piers of the Aramayo and Pisco bridges than HEC – 18 equation, these Optimized ANN estimations were considerably different from the scour measurements made around the previous mentioned piers. | |
dc.format | application/pdf | |
dc.identifier.uri | https://hdl.handle.net/20.500.12996/6864 | |
dc.language.iso | spa | |
dc.publisher | Universidad Nacional Agraria La Molina | |
dc.publisher.country | PE | |
dc.rights | info:eu-repo/semantics/openAccess | |
dc.rights.uri | https://creativecommons.org/licenses/by-nc-nd/4.0/ | |
dc.subject | Puentes | |
dc.subject.ocde | Pendiente | |
dc.title | Redes neuronales artificiales para la estimación de la socavación en pilares de puentes en el Perú | |
dc.type | info:eu-repo/semantics/masterThesis | |
dc.type.version | info:eu-repo/semantics/publishedVersion | |
renati.advisor.dni | 07207616 | |
renati.advisor.orcid | https://orcid.org/0000-0002-8445-8996 | |
renati.author.dni | 42819038 | |
renati.discipline | 522197 | |
renati.juror | Mejía Marcacuzco, Jesús Abel | |
renati.juror | Velásquez Bejarano, Teresa Olinda | |
renati.juror | Apaestegui Castro, James Emiiliano | |
renati.level | https://purl.org/pe-repo/renati/level#maestro | |
renati.type | https://purl.org/pe-repo/renati/type#tesis | |
thesis.degree.discipline | Recursos Hídricos | |
thesis.degree.grantor | Universidad Nacional Agraria La Molina. Escuela de Posgrado | |
thesis.degree.name | Magister Scientiae - Recursos Hídricos |
Files
Original bundle
1 - 3 of 3
Loading...
- Name:
- delgado-caballero-fernando-erdulfo.pdf
- Size:
- 7.17 MB
- Format:
- Adobe Portable Document Format
- Description:
- Texto completo
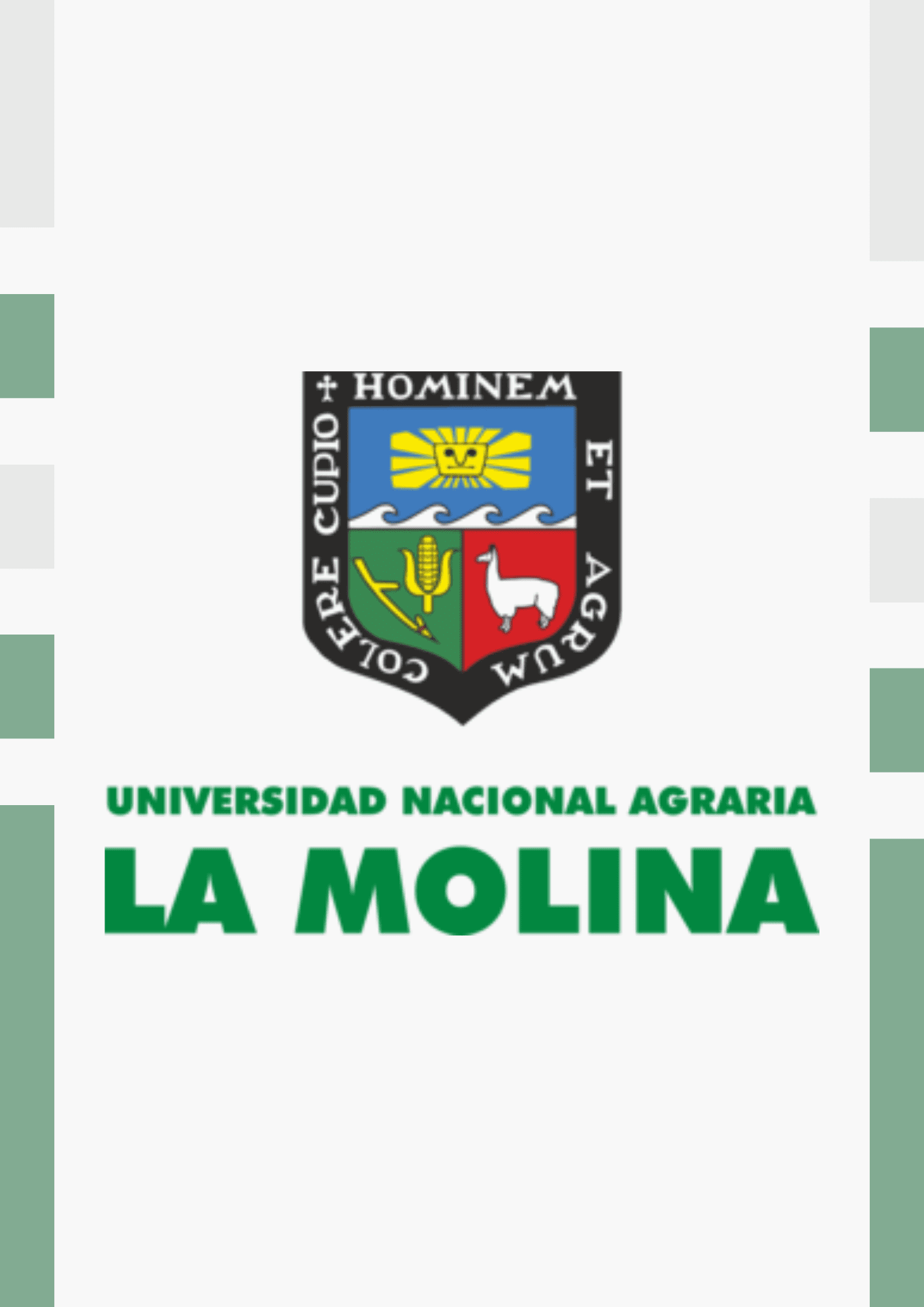
- Name:
- 4. turnitin.pdf
- Size:
- 7.3 MB
- Format:
- Adobe Portable Document Format
- Description:
- Informe originalidad
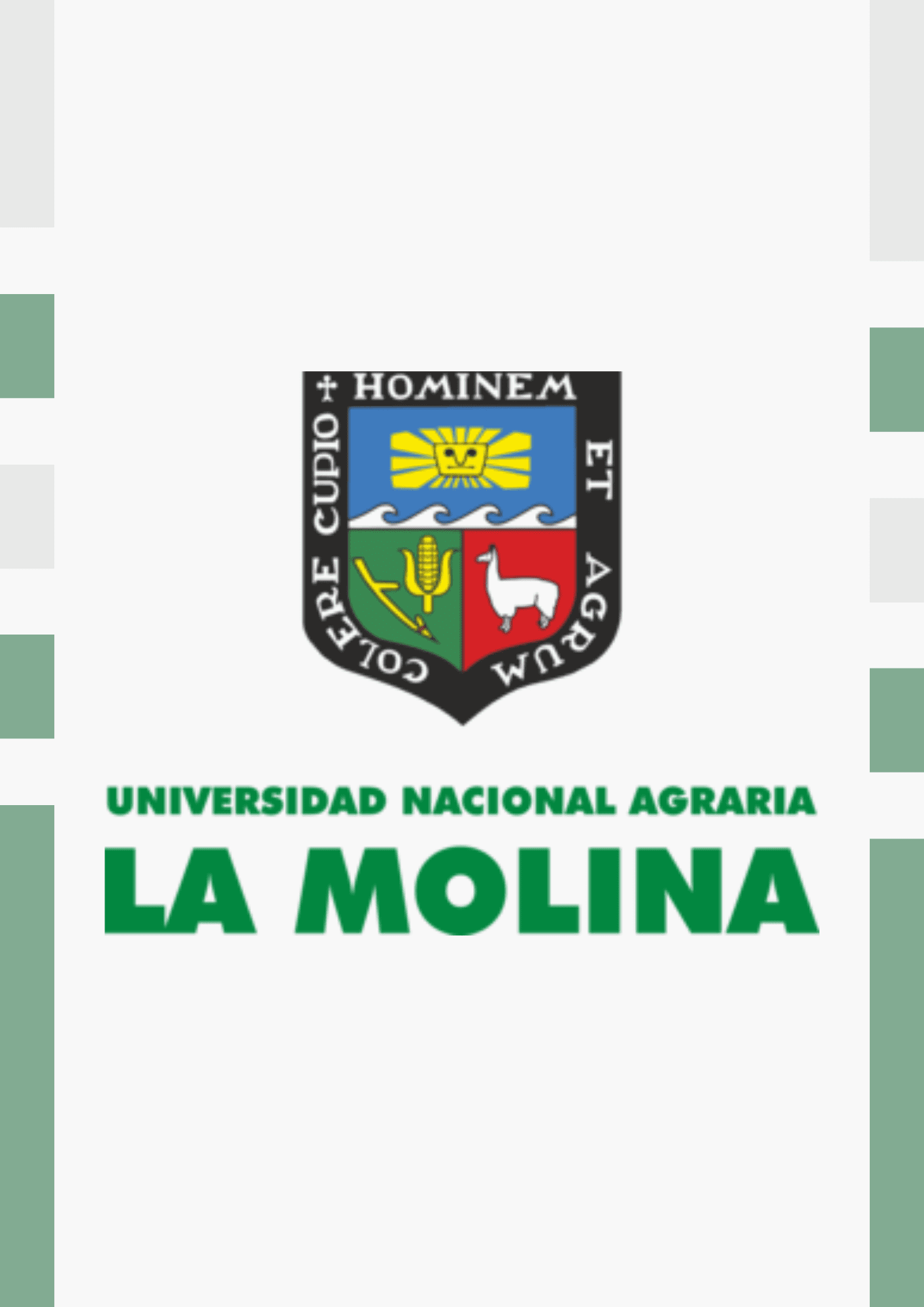
- Name:
- Formato de REPOSITORIO.pdf
- Size:
- 428.01 KB
- Format:
- Adobe Portable Document Format
- Description:
- Autorización
License bundle
1 - 1 of 1
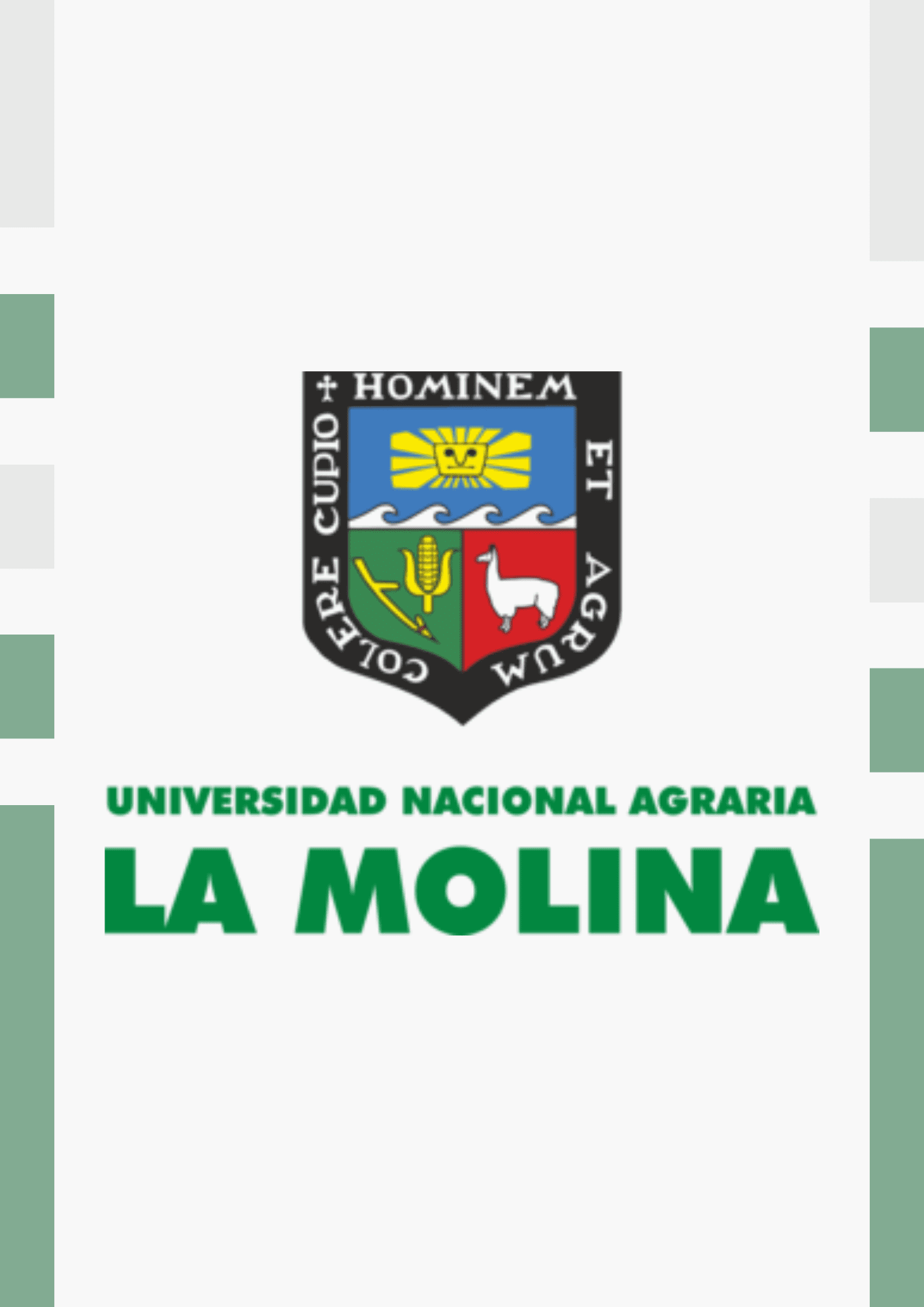
- Name:
- license.txt
- Size:
- 1.63 KB
- Format:
- Item-specific license agreed upon to submission
- Description: